Challenge
New materials for solar panels are critical for advancing solar energy technology, as they have the potential to significantly improve the efficiency, durability, and cost-effectiveness of solar cells. These materials often promise higher conversion efficiencies, better stability under various environmental conditions, and reduced manufacturing costs compared to traditional silicon-based solar panels. However, the development of such materials requires extensive research and experimentation to identify the most promising candidates and optimize their properties. One major challenge hindering progress in this field is the unorganized nature of experimental data, which is scattered across numerous research papers and publications. As researchers investigate a wide range of materials, fabrication techniques, and device architectures, the resulting data is often fragmented and dispersed across different sources
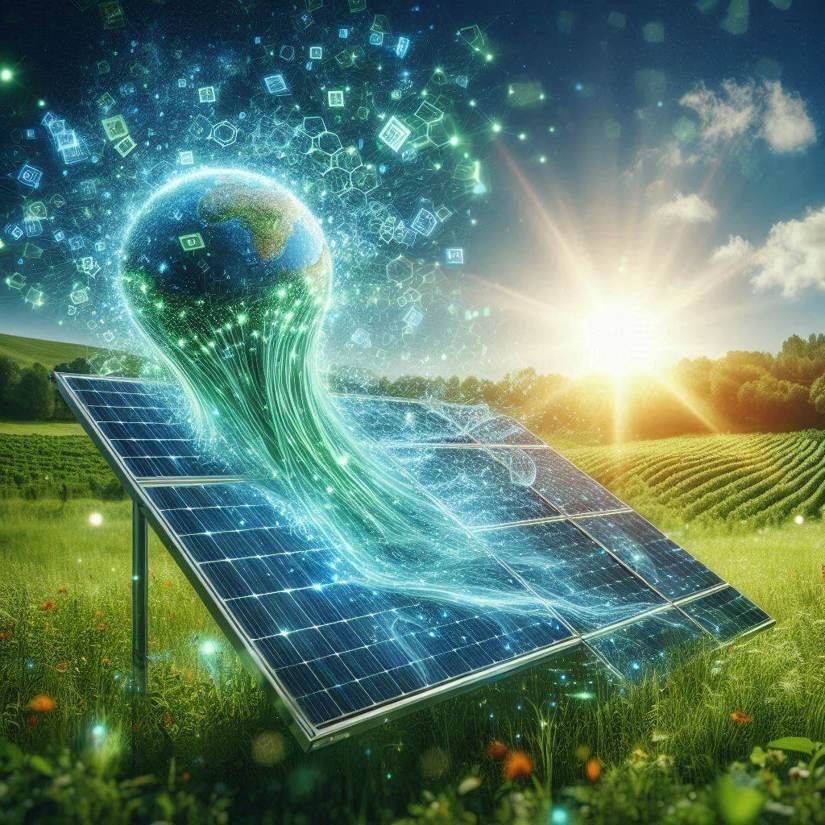
RnD Objectives
In this project, we utilize retrieval-augmented generation techniques based on Large Language Models (LLMs) to extract and organize experimental data related to new solar panel materials from a vast array of sources and research papers. By leveraging the capabilities of LLMs, we aim to efficiently sift through thousands of documents and retrieve relevant information pertaining to the properties, performance, and fabrication processes of novel solar materials. This approach not only facilitates the extraction of key data points but also aids in organizing and structuring the information in a coherent and accessible manner.
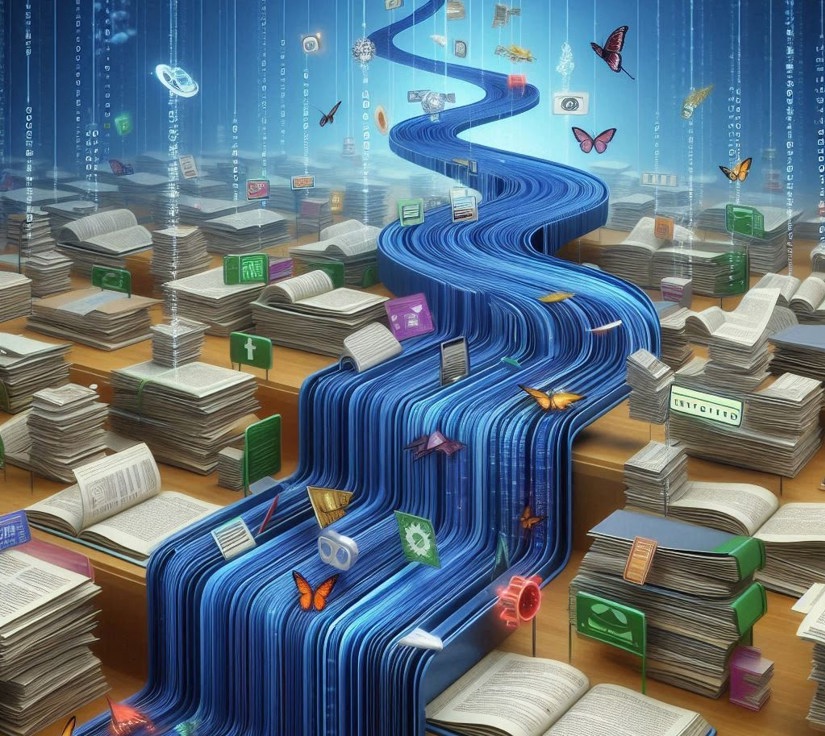
Discovery of novel materials
Share a link using:
https://www.afeka.ac.il/en/industry-relations/research-centers/afeka-interdisciplinary-center-for-social-good-generative-ai/discovery-of-novel-materials/WhatsApp
Facebook
Twitter
Email
https://www.afeka.ac.il/en/industry-relations/research-centers/afeka-interdisciplinary-center-for-social-good-generative-ai/discovery-of-novel-materials/